Amazon AWS Certified Machine Learning - Specialty (MLS-C01) Exam Questions
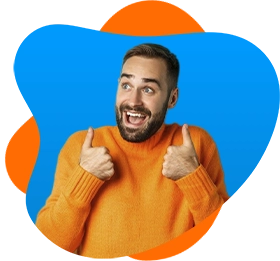
Amazon MLS-C01 Exam Questions, Topics, Explanation and Discussion
Machine Learning Implementation and Operations is a critical domain that focuses on the practical aspects of developing, deploying, and managing machine learning solutions in a production environment. This topic encompasses the entire lifecycle of machine learning projects, from initial design and implementation to ongoing maintenance and optimization. It requires a comprehensive understanding of how to create robust, scalable, and secure machine learning solutions that can effectively address real-world business challenges while leveraging AWS's powerful cloud infrastructure and services.
In the context of the AWS Certified Machine Learning - Specialty exam (MLS-C01), this topic is crucial as it tests candidates' ability to translate theoretical machine learning knowledge into practical, production-ready solutions. The exam syllabus emphasizes not just the technical skills of building machine learning models, but also the operational expertise required to deploy and manage these models effectively in a cloud environment.
The exam will assess candidates' skills through various question types, including:
- Multiple-choice questions that test understanding of best practices for machine learning solution design
- Scenario-based questions that require candidates to recommend appropriate AWS services for specific machine learning challenges
- Problem-solving questions that evaluate the ability to design scalable and resilient machine learning architectures
- Technical questions about security implementation, performance optimization, and deployment strategies
Candidates should be prepared to demonstrate:
- Deep knowledge of AWS machine learning services like SageMaker, Comprehend, and Rekognition
- Understanding of performance optimization techniques
- Ability to implement security best practices
- Skills in designing fault-tolerant and scalable machine learning solutions
- Expertise in model deployment and operationalization
The exam requires a high level of technical proficiency, typically expecting candidates to have hands-on experience with machine learning projects in AWS environments. Candidates should focus on practical skills, understanding how to select the right services, implement security measures, and create robust machine learning solutions that can handle real-world complexity and scale.
Key areas of focus include:
- Performance optimization strategies
- Scalability and availability considerations
- Security implementation
- Model deployment techniques
- Monitoring and management of machine learning solutions
Successful candidates will demonstrate not just theoretical knowledge, but practical skills in implementing end-to-end machine learning solutions that meet complex business requirements while leveraging AWS's comprehensive cloud ecosystem.
Modeling is a critical phase in machine learning that involves transforming business problems into computational solutions and developing predictive or analytical models. It encompasses the entire process of selecting appropriate algorithms, training models with relevant data, optimizing their performance, and rigorously evaluating their effectiveness. The goal of modeling is to create robust, accurate, and generalizable machine learning solutions that can solve real-world problems with high precision and reliability.
In the context of machine learning, modeling requires a systematic approach that involves understanding the underlying business challenge, selecting the most suitable machine learning technique, preparing and preprocessing data, training models, fine-tuning their parameters, and critically assessing their performance across various metrics.
The Modeling topic is a crucial component of the AWS Certified Machine Learning - Specialty exam (MLS-C01), directly aligning with the exam's core competency areas. This section tests candidates' ability to translate business problems into machine learning frameworks, demonstrating comprehensive understanding of model selection, training, optimization, and evaluation techniques. The subtopics cover essential skills that AWS expects machine learning professionals to master, including problem framing, algorithmic selection, model training, hyperparameter tuning, and rigorous model assessment.
Candidates can expect a variety of question types in the exam related to Modeling, including:
- Multiple-choice questions testing theoretical knowledge of machine learning model selection
- Scenario-based questions requiring candidates to recommend appropriate modeling approaches for specific business problems
- Technical questions about hyperparameter optimization strategies
- Conceptual questions exploring model evaluation techniques and performance metrics
The exam will assess candidates' skills at an advanced level, requiring deep understanding of:
- Different machine learning algorithms and their appropriate use cases
- Model training and validation techniques
- Hyperparameter tuning methodologies
- Performance evaluation and model selection criteria
- AWS-specific machine learning services and tools
To excel in this section, candidates should focus on developing a comprehensive understanding of machine learning modeling principles, hands-on experience with AWS machine learning services, and the ability to make strategic decisions about model development and optimization.
Exploratory Data Analysis (EDA) is a critical preliminary step in the machine learning workflow that involves examining and understanding the underlying structure, patterns, and characteristics of a dataset before building predictive models. It serves as a foundational process where data scientists investigate the data's key properties, identify potential issues, and gain insights that will guide subsequent modeling decisions. Through techniques like statistical summarization, data visualization, and preliminary data cleaning, EDA helps researchers understand the relationships between variables, detect anomalies, and prepare data for more advanced machine learning techniques.
In the context of the AWS Certified Machine Learning - Specialty exam (MLS-C01), Exploratory Data Analysis is a crucial component that demonstrates a candidate's ability to effectively prepare and understand complex datasets. The exam syllabus emphasizes the importance of data preparation, feature engineering, and analytical skills that are directly related to EDA principles.
The exam will likely test candidates' knowledge of EDA through various question types, including:
- Multiple-choice questions focusing on data preparation techniques
- Scenario-based questions that require identifying appropriate data cleaning strategies
- Problem-solving questions about feature engineering and data transformation
- Conceptual questions about data visualization and statistical analysis
Candidates should be prepared to demonstrate skills in:
- Identifying and handling missing or inconsistent data
- Performing feature selection and transformation
- Understanding statistical measures and data distributions
- Recognizing appropriate visualization techniques for different data types
- Applying AWS-specific tools like Amazon SageMaker for data exploration
The exam will test not just theoretical knowledge, but practical application of EDA techniques in real-world machine learning scenarios. Candidates should focus on understanding both the conceptual foundations and practical implementation of exploratory data analysis within the AWS ecosystem.
Data Engineering in the context of machine learning is a critical discipline that focuses on preparing, managing, and transforming data to enable effective machine learning model development. It involves creating robust data repositories, implementing efficient data ingestion strategies, and transforming raw data into a format suitable for machine learning algorithms. The goal is to ensure high-quality, clean, and structured data that can be effectively used for training and validating machine learning models.
In AWS, data engineering for machine learning encompasses a wide range of services and techniques that help data scientists and machine learning engineers prepare and process data efficiently. This includes using services like Amazon S3 for data storage, AWS Glue for data transformation, AWS Data Pipeline for data movement, and various ETL (Extract, Transform, Load) tools that facilitate seamless data preparation.
The Data Engineering topic is a crucial component of the AWS Certified Machine Learning - Specialty exam (MLS-C01), directly aligning with the exam's focus on understanding how to prepare and manage data for machine learning workflows. Candidates are expected to demonstrate proficiency in creating data repositories, implementing data ingestion solutions, and executing data transformation techniques using AWS services.
In the actual exam, candidates can expect a variety of question types related to data engineering, including:
- Multiple-choice questions testing knowledge of AWS data storage and processing services
- Scenario-based questions that require selecting the most appropriate data ingestion or transformation strategy
- Questions evaluating understanding of data preprocessing techniques
- Practical problem-solving scenarios involving data pipeline design and implementation
The exam will assess candidates' skills in:
- Selecting appropriate AWS services for data storage and processing
- Understanding data preparation techniques
- Implementing efficient data transformation workflows
- Handling large-scale data engineering challenges
- Ensuring data quality and consistency
Candidates should focus on hands-on experience with AWS services like S3, Glue, Data Pipeline, and Lambda. Practical knowledge of data cleaning, feature engineering, and understanding how to prepare data for different machine learning algorithms will be crucial for success in this section of the exam.
Domain 4: Machine Learning Implementation and Operations focuses on the critical aspects of deploying, managing, and optimizing machine learning solutions in real-world AWS environments. This domain emphasizes the practical skills required to transform machine learning models from theoretical concepts into robust, scalable, and secure production systems. Candidates must understand how to design machine learning solutions that not only perform effectively but also meet enterprise-level requirements for performance, availability, security, and operational efficiency.
The subtopics within this domain cover a comprehensive range of implementation challenges, including solution architecture, service selection, security practices, and operational deployment strategies. Professionals are expected to demonstrate their ability to navigate the complex landscape of machine learning infrastructure, selecting appropriate AWS services, implementing best practices, and ensuring the reliability and scalability of machine learning solutions.
In the AWS Certified Machine Learning - Specialty exam, Domain 4 is crucial as it tests candidates' practical knowledge beyond theoretical machine learning concepts. This domain typically represents approximately 20-25% of the total exam content, highlighting the importance of implementation and operational skills in real-world machine learning scenarios.
The exam syllabus for this domain is closely aligned with industry requirements, focusing on:
- Performance optimization of machine learning solutions
- Scalability and resilience design principles
- Appropriate service and feature selection
- Security implementation in machine learning workflows
- Deployment and operationalization strategies
Candidates can expect a variety of question types in this domain, including:
- Multiple-choice questions testing knowledge of AWS machine learning services
- Scenario-based questions requiring architectural decision-making
- Problem-solving questions about performance and scalability challenges
- Security and compliance-related questions
To excel in this domain, candidates should possess:
- Strong understanding of AWS machine learning and AI services
- Practical experience with cloud infrastructure design
- Knowledge of security best practices
- Ability to evaluate and select appropriate technologies
- Hands-on experience with deployment and monitoring strategies
The skill level required is intermediate to advanced, demanding not just theoretical knowledge but practical implementation skills. Candidates should be prepared to demonstrate their ability to design, deploy, and manage machine learning solutions that meet complex enterprise requirements while leveraging AWS's comprehensive machine learning ecosystem.
Domain 3: Modeling is a critical section of the AWS Certified Machine Learning - Specialty exam that focuses on the core technical skills required to develop and implement machine learning solutions. This domain covers the entire lifecycle of machine learning model development, from problem framing to model selection, training, optimization, and evaluation. Candidates are expected to demonstrate a comprehensive understanding of how to transform business challenges into machine learning problems, select appropriate algorithms, train models effectively, and critically assess their performance.
The modeling domain represents the practical application of machine learning techniques, emphasizing the candidate's ability to make informed decisions throughout the model development process. It requires a deep understanding of various machine learning algorithms, their strengths, limitations, and appropriate use cases across different business scenarios.
The relationship between this domain and the exam syllabus is fundamental. The AWS Certified Machine Learning - Specialty exam (MLS-C01) is designed to validate an individual's ability to design, implement, deploy, and maintain machine learning solutions on AWS. The Modeling domain specifically tests candidates' technical proficiency in translating business problems into machine learning challenges and executing the entire model development lifecycle.
Candidates can expect a variety of question types in this domain, including:
- Multiple-choice questions that assess understanding of machine learning problem framing
- Scenario-based questions requiring candidates to select the most appropriate model for a given business problem
- Technical questions about model training techniques and hyperparameter optimization
- Analytical questions focused on model evaluation metrics and performance assessment
The exam will test candidates on several key skills:
- Ability to identify suitable machine learning approaches for different business scenarios
- Understanding of various machine learning algorithms and their appropriate applications
- Proficiency in model training techniques
- Knowledge of hyperparameter tuning methods
- Skill in evaluating model performance using appropriate metrics
To excel in this domain, candidates should have hands-on experience with machine learning model development, a strong theoretical understanding of different algorithms, and practical knowledge of AWS machine learning services and tools. The exam requires a mix of theoretical knowledge and practical application, with a focus on making informed, strategic decisions in machine learning solution design.
Preparation should include:
- Comprehensive study of machine learning algorithms
- Practical experience with model development
- Understanding of AWS-specific machine learning services
- Practice with real-world scenario analysis
- Familiarity with model evaluation techniques
Exploratory Data Analysis (EDA) is a critical phase in the machine learning workflow that involves examining and understanding the underlying characteristics, patterns, and potential issues within a dataset before building predictive models. This process is essential for data scientists and machine learning practitioners to gain insights, identify data quality problems, and prepare data for effective model development. EDA encompasses a range of techniques including data cleaning, feature engineering, statistical analysis, and data visualization that help transform raw data into meaningful information.
In the context of the AWS Certified Machine Learning - Specialty exam, Domain 2 focuses on the crucial skills required to manipulate and prepare data effectively. Candidates must demonstrate their ability to sanitize datasets, engineer relevant features, and create meaningful visualizations that reveal important insights about the data. This domain tests a candidate's proficiency in handling real-world data challenges and preparing datasets for machine learning model development.
The exam syllabus for this domain emphasizes the following key relationships:
- Direct alignment with practical machine learning data preparation techniques
- Understanding of AWS-specific tools and services for data analysis
- Comprehensive approach to data preprocessing and feature engineering
Candidates can expect the following types of exam questions for this domain:
- Multiple-choice questions testing theoretical knowledge of data preparation techniques
- Scenario-based questions that require identifying appropriate data cleaning strategies
- Problem-solving questions about feature engineering and selection
- Visualization and interpretation challenges that assess understanding of data characteristics
The exam will test candidates' skills at an intermediate to advanced level, requiring:
- Deep understanding of data preprocessing techniques
- Ability to identify and handle missing or corrupted data
- Proficiency in feature transformation and selection
- Knowledge of statistical techniques for data analysis
- Familiarity with AWS services like Amazon SageMaker for data preparation
Key skills to focus on include:
- Data cleaning and normalization
- Handling categorical and numerical features
- Dimensionality reduction techniques
- Statistical analysis and data visualization
- Understanding of overfitting and feature selection strategies
Recommended preparation strategies include practicing with real-world datasets, understanding AWS machine learning tools, and developing a systematic approach to data exploration and preprocessing.
Domain 1: Data Engineering is a critical component of the AWS Certified Machine Learning - Specialty exam, focusing on the foundational aspects of preparing and managing data for machine learning workflows. This domain emphasizes the importance of creating robust data repositories, implementing efficient data ingestion strategies, and developing effective data transformation techniques that enable high-quality machine learning model development.
The data engineering domain covers the essential skills required to handle complex data challenges in machine learning projects, ensuring that data is properly collected, stored, processed, and prepared for advanced analytics and model training. Candidates must demonstrate proficiency in selecting appropriate AWS services and implementing best practices for data management and preprocessing.
The subtopics in this domain (1.1 Create data repositories for machine learning, 1.2 Identify and implement a data-ingestion solution, and 1.3 Identify and implement a data-transformation solution) are directly aligned with the exam syllabus and test a candidate's ability to design and implement comprehensive data engineering solutions using AWS technologies.
Relationship to Exam Syllabus:
- Covers approximately 20% of the total exam content
- Tests practical knowledge of AWS data services like S3, Glue, Lake Formation, and Redshift
- Evaluates understanding of data preparation techniques for machine learning
Exam Question Types and Skills:
- Multiple-choice questions testing theoretical knowledge of data engineering concepts
- Scenario-based questions requiring candidates to select appropriate AWS services for specific data challenges
- Practical problem-solving questions about data ingestion, transformation, and repository design
- Questions assessing knowledge of:
- Data storage architectures
- ETL (Extract, Transform, Load) processes
- Data preprocessing techniques
- AWS data service capabilities
Skill Level Requirements:
- Intermediate to advanced understanding of AWS data services
- Ability to design scalable and efficient data pipelines
- Knowledge of data cleaning, normalization, and feature engineering techniques
- Practical experience with real-world data engineering challenges
Key Preparation Strategies:
- Study AWS documentation thoroughly
- Practice hands-on labs and workshops
- Understand data preprocessing techniques
- Learn best practices for machine learning data preparation