HP Using HPE AI and Machine Learning (HPE2-N69) Exam Questions
As you gear up to conquer the HP Using HPE AI and Machine Learning HPE2-N69 exam, having a solid understanding of the official syllabus, engaging in discussions, familiarizing yourself with the expected exam format, and practicing with sample questions are vital. Our platform offers a wealth of resources to help you excel in this certification, guiding you through the intricacies of AI and machine learning technologies. Whether you are aiming to enhance your expertise for career advancement or seeking new opportunities in the tech industry, mastering this exam could be your key to success. Dive into the world of HP Using HPE AI and Machine Learning with confidence and let your skills shine brightly in the realm of artificial intelligence.
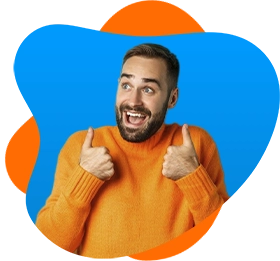
HPE2-N69 Exam Questions, Topics, Explanation and Discussion
Engaging with customers is a crucial aspect of implementing AI and Machine Learning solutions in an enterprise environment. This topic focuses on understanding customer needs, pain points, and business objectives to effectively propose and implement HPE AI and ML solutions. It involves active listening, asking probing questions, and gathering relevant information about the customer's current infrastructure, data landscape, and desired outcomes. Additionally, it covers the ability to articulate the value proposition of HPE AI and ML offerings, addressing potential concerns, and aligning solutions with the customer's specific requirements and goals.
This topic is fundamental to the HPE2-N69 exam as it forms the foundation for successful AI and ML implementations. Understanding how to engage with customers effectively is essential for tailoring HPE solutions to meet specific business needs and ensuring customer satisfaction. It relates closely to other exam topics such as identifying use cases, designing solutions, and overcoming implementation challenges. Mastery of this topic demonstrates the candidate's ability to bridge the gap between technical knowledge and practical application in real-world scenarios.
Candidates can expect the following types of questions on this topic:
- Multiple-choice questions testing knowledge of effective customer engagement techniques and best practices
- Scenario-based questions presenting a customer situation and asking candidates to identify the most appropriate approach or solution
- Questions assessing the ability to match customer requirements with specific HPE AI and ML offerings
- True/false or multiple-choice questions on common customer pain points and how to address them
- Questions evaluating the candidate's understanding of how to articulate the value proposition of HPE AI and ML solutions to different stakeholders
The depth of knowledge required will range from recall of key concepts to application of principles in complex customer scenarios. Candidates should be prepared to demonstrate their understanding of customer engagement strategies and their ability to apply this knowledge in practical situations.
The HPE Machine Learning Development Environment is a comprehensive platform designed to streamline the process of developing, training, and deploying machine learning models. It provides data scientists and ML engineers with a collaborative workspace that includes tools for data preparation, model development, and experiment tracking. Key features of this environment include support for popular ML frameworks like TensorFlow and PyTorch, integration with version control systems, and the ability to scale computations across distributed resources. Users can leverage pre-built templates and workflows to accelerate their development process, while also having the flexibility to customize their environment as needed.
This topic is crucial to the HPE2-N69 exam as it focuses on the practical application of AI and machine learning technologies within the HPE ecosystem. Understanding how to effectively use the HPE Machine Learning Development Environment is essential for candidates aiming to demonstrate their proficiency in implementing and managing AI/ML solutions using HPE tools. This knowledge directly aligns with the exam's objective of assessing a candidate's ability to leverage HPE's AI and ML capabilities in real-world scenarios.
Candidates can expect a variety of question types on this topic in the actual exam:
- Multiple-choice questions testing knowledge of specific features and capabilities of the HPE Machine Learning Development Environment
- Scenario-based questions that require candidates to identify the appropriate use of the environment in given situations
- Hands-on simulation questions where candidates might need to demonstrate their ability to navigate and use key features of the environment
- Questions focusing on best practices for collaboration, version control, and scaling ML workloads within the environment
- Comparative questions that ask candidates to differentiate between the HPE Machine Learning Development Environment and other ML platforms or tools
The depth of knowledge required will range from basic understanding of the environment's components to more advanced concepts related to optimizing ML workflows and leveraging distributed computing resources. Candidates should be prepared to not only recall information but also apply their knowledge to solve practical problems and make informed decisions about using the HPE Machine Learning Development Environment in various scenarios.
The architecture for HPE Machine Learning Development solutions typically consists of several key components designed to support the entire machine learning lifecycle. This includes data preparation, model development, training, and deployment. The architecture often incorporates HPE's high-performance computing (HPC) infrastructure, such as HPE Apollo systems, which provide the necessary computational power for complex ML workloads. It also includes software components like HPE Ezmeral MLOps, which facilitates model management, deployment, and monitoring. The architecture is designed to be scalable and flexible, allowing organizations to start small and grow their ML capabilities as needed. It often integrates with popular open-source ML frameworks and tools, ensuring compatibility with existing workflows and skillsets.
This topic is crucial to the HPE2-N69 exam as it forms the foundation for understanding how HPE's ML solutions are structured and implemented. It relates directly to the exam's focus on HPE's AI and Machine Learning offerings, and understanding this architecture is key to grasping how HPE supports the entire ML lifecycle. This knowledge is essential for candidates who need to design, implement, or support HPE ML solutions in real-world scenarios.
Candidates can expect several types of questions on this topic in the exam:
- Multiple-choice questions asking to identify key components of the HPE ML Development architecture
- Scenario-based questions where candidates need to recommend appropriate architectural elements for specific ML use cases
- Questions about the benefits and features of different components within the architecture
- Matching questions linking architectural components to their functions or characteristics
- True/False questions about the capabilities and limitations of the HPE ML Development architecture
The depth of knowledge required will likely include understanding the purpose and function of each major component, how they interact, and how they support different stages of the ML lifecycle. Candidates should be prepared to apply this knowledge to real-world scenarios and demonstrate an understanding of how the architecture supports scalability, performance, and integration with existing tools and frameworks.
Articulating the business case for HPE Machine Learning Development solutions involves understanding and communicating the value proposition of HPE's AI and ML offerings to potential clients. This includes highlighting the benefits of HPE's hardware and software solutions designed specifically for ML workloads, such as improved performance, scalability, and efficiency. Key aspects include discussing how HPE's solutions can accelerate time-to-insight, reduce operational costs, and enable organizations to derive more value from their data. Additionally, it's important to emphasize HPE's expertise in enterprise-grade infrastructure and how it translates to robust, secure, and reliable ML development environments.
This topic is crucial to the overall HPE2-N69 exam as it forms the foundation for understanding HPE's position in the AI and ML market. Candidates need to be able to effectively communicate the advantages of HPE's solutions to potential customers, which requires a deep understanding of both the technical aspects and the business benefits. This knowledge is essential for roles involving sales, consulting, or solution architecture in the HPE AI and ML ecosystem.
Candidates can expect various types of questions on this topic in the exam:
- Multiple-choice questions testing knowledge of specific benefits of HPE ML Development solutions
- Scenario-based questions where candidates must identify the most appropriate HPE solution for a given business problem
- Questions requiring candidates to match HPE products or features with their corresponding business benefits
- Case study analysis questions where candidates must articulate the business case for implementing HPE ML solutions in a specific industry or use case
The depth of knowledge required will range from recall of key features and benefits to the ability to synthesize information and apply it to real-world scenarios. Candidates should be prepared to demonstrate a comprehensive understanding of HPE's ML Development solutions and their business impact.
Machine learning (ML) and deep learning (DL) fundamentals are essential concepts in artificial intelligence. ML focuses on algorithms that enable computers to learn from and make predictions or decisions based on data, without being explicitly programmed. It encompasses various techniques such as supervised learning, unsupervised learning, and reinforcement learning. Deep learning, a subset of ML, utilizes artificial neural networks with multiple layers to model and process complex patterns in data. DL has shown remarkable success in areas like image and speech recognition, natural language processing, and autonomous systems. Understanding these fundamentals includes knowledge of key algorithms, model architectures, training processes, and evaluation metrics.
This topic is crucial to the HPE2-N69 exam as it forms the foundation for understanding and implementing AI and ML solutions using HPE technologies. The exam likely covers how these fundamental concepts are applied in HPE's AI and ML offerings, including hardware and software solutions. Candidates should be able to demonstrate a solid grasp of ML and DL principles to effectively design, deploy, and manage AI systems within HPE environments.
Candidates can expect a variety of question types on this topic, including:
- Multiple-choice questions testing knowledge of ML and DL terminology, algorithms, and concepts
- Scenario-based questions requiring application of ML/DL principles to real-world problems
- Questions comparing different ML/DL approaches and their suitability for specific use cases
- Questions on selecting appropriate ML/DL techniques for given datasets or business objectives
- Questions about interpreting ML/DL model outputs and performance metrics
The depth of knowledge required will likely range from basic definitions to more advanced understanding of how these concepts are implemented in HPE's AI and ML solutions.