IBM AI Enterprise Workflow V1 Data Science Specialist (C1000-059) Exam Questions
Are you ready to take your career to the next level with the IBM AI Enterprise Workflow V1 Data Science Specialist certification? Dive deep into the official syllabus, engage in discussions around key topics, familiarize yourself with the expected exam format, and sharpen your skills with sample questions. At our platform, we provide you with the essential tools to prepare for success in the C1000-059 exam. Whether you are aspiring to become a Data Scientist, Machine Learning Engineer, or AI Specialist, this certification is your gateway to unlocking new opportunities in the field of AI and data science. Stay ahead of the curve and join a community of like-minded professionals dedicated to mastering the latest technologies and advancing their careers. Get started on your path to success today!
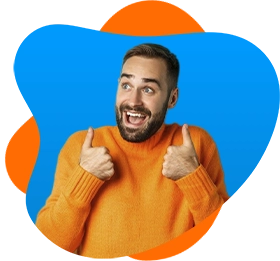
IBM C1000-059 Exam Questions, Topics, Explanation and Discussion
Section 8: Technology Stack for Data Science and AI focuses on the various tools, platforms, and technologies used in the field of data science and artificial intelligence. This section covers essential components such as programming languages (e.g., Python, R), data manipulation and analysis libraries (e.g., pandas, NumPy), machine learning frameworks (e.g., scikit-learn, TensorFlow), and big data processing tools (e.g., Apache Spark). It also includes topics related to data storage and management systems, cloud computing platforms, and visualization tools. Candidates are expected to understand the strengths and use cases of different technologies, as well as how they integrate within the AI enterprise workflow.
This topic is crucial to the IBM AI Enterprise Workflow V1 Data Science Specialist certification exam as it forms the foundation for implementing and managing AI solutions in enterprise environments. Understanding the technology stack is essential for data scientists to effectively design, develop, and deploy AI models and applications. It relates closely to other exam sections, such as data preparation, model development, and deployment, as the choice of tools and technologies directly impacts these processes. Proficiency in this area demonstrates a candidate's ability to select and utilize appropriate technologies for various data science and AI tasks within an enterprise context.
Candidates can expect the following types of questions on this topic:
- Multiple-choice questions testing knowledge of specific tools and their primary functions (e.g., "Which of the following is primarily used for distributed data processing?")
- Scenario-based questions requiring candidates to select the most appropriate technology for a given use case (e.g., "A company needs to process large volumes of streaming data. Which technology would be most suitable?")
- Questions comparing and contrasting different technologies (e.g., "What are the key differences between TensorFlow and PyTorch?")
- Questions about integration and compatibility between different tools in the data science ecosystem
- Practical questions on how to use specific libraries or frameworks to accomplish common data science tasks
The depth of knowledge required will range from basic understanding of tool capabilities to more advanced concepts of how these technologies fit into the overall AI enterprise workflow. Candidates should be prepared to demonstrate both theoretical knowledge and practical application of the various components in the data science and AI technology stack.
Section 7: Deployment of AI models focuses on the crucial final stage of the AI development lifecycle. This section covers the process of taking a trained and validated AI model and integrating it into a production environment where it can provide value to end-users or other systems. Key sub-topics include containerization using technologies like Docker, orchestration with Kubernetes, and deployment strategies such as blue-green or canary deployments. Candidates should understand how to package models with their dependencies, ensure scalability and performance in production, and implement monitoring and logging for deployed models. Additionally, this section may cover topics like model versioning, A/B testing, and the challenges of maintaining model accuracy over time in real-world scenarios.
This topic is critical to the overall IBM AI Enterprise Workflow V1 Data Science Specialist certification as it represents the culmination of the AI development process. While earlier sections of the exam focus on data preparation, model development, and validation, this section tests a candidate's ability to bridge the gap between a successful model in a development environment and a robust, scalable solution in production. Understanding deployment is essential for data scientists working in enterprise environments, as it ensures that their work can deliver tangible business value and integrate smoothly with existing systems and processes.
Candidates can expect a variety of question types on this topic in the actual exam:
- Multiple-choice questions testing knowledge of deployment concepts, tools, and best practices
- Scenario-based questions that present a specific deployment challenge and ask candidates to choose the best solution or identify potential issues
- Questions that require interpreting logs or metrics from a deployed model to diagnose problems or suggest improvements
- Tasks involving selecting appropriate deployment strategies for different use cases or business requirements
- Questions about security considerations and best practices for deploying AI models in enterprise environments
The depth of knowledge required will range from basic familiarity with deployment concepts to the ability to analyze complex scenarios and make informed decisions about deployment strategies and troubleshooting.
Section 6: Evaluation of AI models focuses on assessing the performance and effectiveness of artificial intelligence models in enterprise workflows. This section covers various evaluation metrics and techniques used to measure model accuracy, precision, recall, and F1 score. It also delves into the interpretation of confusion matrices, ROC curves, and AUC values. Additionally, candidates should understand the importance of model validation, cross-validation techniques, and how to handle overfitting and underfitting. The section may also touch upon the evaluation of different types of AI models, including classification, regression, and clustering models, as well as the assessment of model fairness and bias.
This topic is crucial to the overall IBM AI Enterprise Workflow V1 Data Science Specialist exam as it ensures that candidates can effectively assess and validate AI models in real-world enterprise scenarios. Understanding model evaluation is essential for making informed decisions about model deployment, refinement, and ongoing maintenance. It relates closely to other sections of the exam, such as data preparation, feature engineering, and model selection, as evaluation results often inform iterative improvements in these areas.
Candidates can expect a variety of question types on this topic in the actual exam:
- Multiple-choice questions testing knowledge of evaluation metrics and their appropriate use cases
- Scenario-based questions requiring interpretation of evaluation results and recommendation of next steps
- Calculation questions involving the computation of specific metrics given a set of model outputs
- Questions on selecting appropriate evaluation techniques for different types of AI models
- Case studies requiring candidates to analyze model performance and suggest improvements
- Questions on identifying and addressing bias in model evaluation
The depth of knowledge required will range from basic understanding of evaluation concepts to the ability to apply these concepts in complex enterprise scenarios. Candidates should be prepared to not only recall definitions but also demonstrate practical application of evaluation techniques in various AI workflows.
Section 5 of the IBM AI Enterprise Workflow V1 Data Science Specialist exam focuses on the practical application of Data Science and AI techniques and models. This section covers the implementation of various machine learning algorithms, deep learning models, and AI techniques to solve real-world business problems. Candidates are expected to demonstrate their understanding of model selection, hyperparameter tuning, and performance evaluation. Additionally, this section may include topics such as feature engineering, dimensionality reduction, and handling imbalanced datasets. The application of these techniques is often contextualized within specific industry use cases, emphasizing the importance of aligning AI solutions with business objectives.
This topic is crucial to the overall exam as it represents the practical implementation of the theoretical concepts covered in earlier sections. It tests the candidate's ability to apply their knowledge in real-world scenarios, which is essential for a Data Science Specialist working in an enterprise environment. The application of Data Science and AI techniques directly relates to the core competencies required for the certification, demonstrating the candidate's readiness to tackle complex data-driven problems in a business context.
Candidates can expect a variety of question types on this topic, including:
- Multiple-choice questions testing knowledge of specific algorithms and their appropriate use cases
- Scenario-based questions requiring candidates to select the most suitable model or technique for a given business problem
- Questions on interpreting model outputs and performance metrics
- Code snippet analysis to identify errors or suggest improvements in model implementation
- Case studies requiring candidates to propose a complete workflow for solving a complex data science problem
The depth of knowledge required will be substantial, with questions often requiring candidates to not only identify the correct approach but also justify their choices based on the given context. Candidates should be prepared to demonstrate a thorough understanding of the strengths and limitations of various techniques, as well as their practical applications in an enterprise setting.
Data preparation techniques in Data Science and AI are crucial steps in the data analysis process. This section covers various methods to clean, transform, and organize data for effective analysis and model building. Key sub-topics include data cleaning (handling missing values, outliers, and inconsistencies), data transformation (normalization, standardization, encoding categorical variables), feature engineering (creating new features, dimensionality reduction), and data integration (merging datasets, handling time-series data). The section also emphasizes the importance of understanding data quality issues and implementing appropriate strategies to address them, ensuring that the data is suitable for machine learning algorithms and AI applications.
This topic is fundamental to the IBM AI Enterprise Workflow V1 Data Science Specialist certification exam as it forms the foundation for all subsequent data analysis and model development tasks. Proper data preparation is essential for building accurate and reliable AI models. It directly relates to other sections of the exam, such as exploratory data analysis, model selection, and deployment, as the quality of prepared data significantly impacts the performance and reliability of AI solutions in enterprise environments.
Candidates can expect a variety of question types on this topic in the actual exam:
- Multiple-choice questions testing knowledge of different data preparation techniques and their appropriate use cases
- Scenario-based questions requiring candidates to identify the most suitable data preparation approach for a given business problem
- Code interpretation questions where candidates need to analyze code snippets related to data cleaning or transformation
- Short answer questions asking candidates to explain the impact of specific data preparation techniques on model performance
- Case study questions requiring candidates to design a comprehensive data preparation strategy for a complex enterprise AI project
The depth of knowledge required will range from basic understanding of concepts to practical application in real-world scenarios. Candidates should be prepared to demonstrate their ability to select and apply appropriate data preparation techniques based on the characteristics of the data and the requirements of the AI project.
Data understanding techniques in Data Science and AI are crucial components of the data analysis process. This section focuses on methods used to gain insights into datasets, including exploratory data analysis (EDA), statistical analysis, and data visualization. Key sub-topics include descriptive statistics, correlation analysis, and data profiling. Candidates should be familiar with techniques for identifying patterns, anomalies, and relationships within datasets, as well as methods for handling missing data and outliers. Understanding data distributions, variable types, and data quality issues is also essential. Additionally, this section may cover tools and libraries commonly used for data understanding, such as pandas, matplotlib, and seaborn in Python.
This topic is fundamental to the IBM AI Enterprise Workflow V1 Data Science Specialist certification as it forms the foundation for effective data analysis and model development. Data understanding techniques are critical for making informed decisions throughout the AI workflow, from data preparation to model selection and evaluation. A solid grasp of these concepts enables data scientists to extract meaningful insights from raw data, identify potential issues early in the process, and make data-driven decisions. This knowledge is essential for success in subsequent stages of the AI workflow, such as feature engineering, model development, and result interpretation.
Candidates can expect a variety of question types on this topic in the C1000-059 exam:
- Multiple-choice questions testing knowledge of specific data understanding techniques and their applications
- Scenario-based questions requiring candidates to select appropriate methods for analyzing given datasets
- Interpretation questions based on data visualizations or statistical outputs
- Questions on identifying data quality issues and proposing solutions
- Code snippet questions related to implementing data understanding techniques using Python libraries
The depth of knowledge required will range from basic concept recognition to practical application and interpretation of results. Candidates should be prepared to demonstrate their understanding of when and how to apply various data understanding techniques in real-world scenarios.
Section 2: Applications of Data Science and AI in Business focuses on the practical implementation of data science and artificial intelligence techniques in various business contexts. This section covers key areas such as customer segmentation, predictive maintenance, fraud detection, and recommendation systems. Candidates are expected to understand how these applications can drive business value, improve decision-making processes, and enhance operational efficiency. The section also delves into the ethical considerations and challenges associated with implementing AI solutions in business environments, including data privacy, bias mitigation, and responsible AI practices.
This topic is crucial to the overall IBM AI Enterprise Workflow V1 Data Science Specialist certification exam as it bridges the gap between theoretical knowledge and real-world applications. It demonstrates the candidate's ability to translate data science concepts into tangible business solutions, which is a key skill for professionals in this field. Understanding the applications of data science and AI in business contexts allows candidates to better align their technical expertise with organizational goals and stakeholder needs.
Candidates can expect a variety of question types on this topic, including:
- Multiple-choice questions testing knowledge of specific AI applications and their benefits in business settings
- Scenario-based questions requiring candidates to identify the most appropriate data science or AI solution for a given business problem
- Case study questions that assess the candidate's ability to evaluate the potential impact and challenges of implementing AI solutions in real-world business scenarios
- Questions on ethical considerations and best practices for responsible AI implementation in business contexts
- Conceptual questions on the integration of AI solutions with existing business processes and systems
The depth of knowledge required will range from basic understanding of AI applications to more advanced concepts involving the strategic implementation and management of AI solutions in enterprise environments.
Section 1 of the IBM AI Enterprise Workflow V1 Data Science Specialist exam focuses on the fundamental scientific, mathematical, and technical concepts essential for Data Science and AI. This section covers a range of topics, including probability theory, statistics, linear algebra, calculus, and optimization techniques. Candidates are expected to demonstrate a solid understanding of these foundational concepts and their applications in data science and AI workflows. Additionally, this section may include topics related to programming languages commonly used in data science, such as Python or R, as well as basic data structures and algorithms.
This topic is crucial to the overall exam as it forms the basis for more advanced concepts and techniques in data science and AI. A strong grasp of these fundamentals is essential for effectively implementing and understanding complex machine learning algorithms, statistical analyses, and AI models. The scientific and mathematical principles covered in this section underpin many of the practical applications and methodologies discussed in later sections of the exam, making it a critical component of the certification.
Candidates can expect a variety of question types on this topic, including:
- Multiple-choice questions testing theoretical knowledge of mathematical and statistical concepts
- Scenario-based questions requiring the application of scientific principles to real-world data science problems
- Short coding exercises or code interpretation questions related to basic programming concepts
- Questions involving the interpretation of mathematical formulas or statistical outputs
- Problem-solving questions that require candidates to apply optimization techniques or probability theory
The depth of knowledge required for this section is typically at an intermediate level, assuming candidates have a background in mathematics, statistics, or a related field. Questions may range from straightforward concept checks to more complex scenarios requiring the integration of multiple principles.